Exploring Generative AI: Revolutionizing Industries with Artificial Creativity
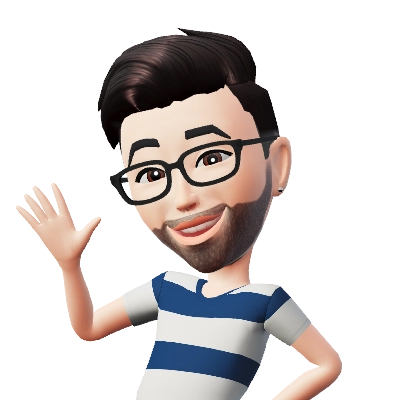
Anirban Das
4 mins read
Table of Contents
IntroductionWhat is Generative AI?Key Technologies Behind Generative AIApplications of Generative AI1. Content CreationIntroduction
Generative AI has been making waves across various industries, transforming how we approach creativity, problem-solving, and automation. Unlike traditional AI models that are designed to classify or predict data, generative AI models can create new content, be it text, images, music, or even entire virtual worlds. In this blog, we will explore what generative AI is, its underlying technologies, and some of its most compelling applications.
What is Generative AI?
Generative AI refers to a subset of artificial intelligence that focuses on generating new content based on the data it has been trained on. The most well-known type of generative AI is the Generative Adversarial Network (GAN), introduced by Ian Goodfellow and his colleagues in 2014. GANs consist of two neural networks: the generator, which creates new data, and the discriminator, which evaluates the data's authenticity. Through this adversarial process, the generator improves its ability to create realistic content over time.
Another prominent model is the Transformer, particularly its variant GPT (Generative Pre-trained Transformer), which excels in generating coherent and contextually relevant text. OpenAI's GPT-4, for instance, can produce human-like text, answer questions, and even write code.
Key Technologies Behind Generative AI
Generative Adversarial Networks (GANs): GANs are designed to create realistic images, videos, and audio by pitting two networks against each other. The generator creates content, while the discriminator attempts to detect if the content is real or generated.
Variational Autoencoders (VAEs): VAEs are used for generating new data points by learning the distribution of the input data. They are often employed in creating variations of images and other types of data.
Transformers: The Transformer architecture has revolutionized natural language processing (NLP). Models like GPT-4 leverage massive amounts of text data to generate human-like text and perform a variety of language tasks.
Applications of Generative AI
1. Content Creation
Generative AI is transforming content creation across multiple domains. In writing, AI tools can generate articles, stories, and poetry. For instance, tools like Jasper and Writesonic help marketers and writers produce high-quality content quickly. In visual arts, AI can create stunning artworks, from digital paintings to intricate designs, as seen with DALL-E, an AI model by OpenAI that generates images from textual descriptions.
2. Entertainment and Media
In the entertainment industry, generative AI is being used to create music, video game content, and even entire movie scripts. AI composers like AIVA create original music compositions, while gaming companies use AI to generate complex and dynamic game environments. The potential for personalized and immersive experiences is vast, opening up new creative possibilities.
3. Healthcare
Generative AI is making significant strides in healthcare, particularly in drug discovery and medical imaging. AI models can generate potential drug molecules, speeding up the discovery process. In medical imaging, generative models enhance image resolution and aid in the diagnosis of diseases by generating detailed images from less clear originals.
4. Fashion and Design
In fashion, generative AI assists designers by creating new clothing patterns and styles. It can analyze trends and generate designs that align with current fashion sensibilities. Companies like Stitch Fix use AI to recommend personalized clothing choices based on customer preferences.
5. Business and Finance
Generative AI is also making an impact in the business world. It is used to generate synthetic data for training machine learning models, helping to overcome data scarcity issues. In finance, AI models generate realistic financial data, aiding in risk assessment and financial forecasting.
Ethical Considerations
While generative AI offers tremendous potential, it also raises ethical concerns. The ability to create highly realistic content can be misused for generating deepfakes or misleading information. Ensuring the ethical use of generative AI involves developing robust detection methods, setting regulatory frameworks, and promoting transparency in AI-generated content.
Conclusion
Generative AI represents a significant leap forward in the capabilities of artificial intelligence. Its applications are vast and varied, impacting numerous industries and transforming how we create and interact with content. As the technology continues to evolve, it will be crucial to address the ethical challenges it presents while harnessing its potential to drive innovation and creativity.
Generative AI is not just a technological advancement; it is a paradigm shift in how we approach creativity and problem-solving in the digital age. Embracing this change will undoubtedly lead to exciting and uncharted territories in the future of AI.